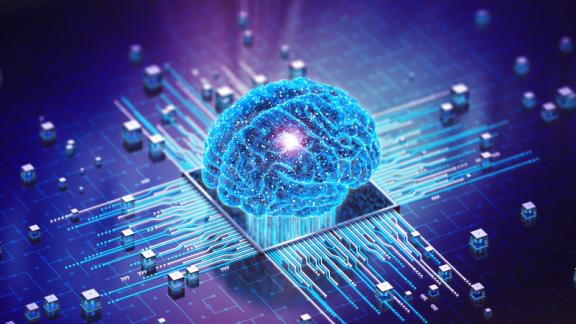
Background
The rapid expansion of artificial intelligence (AI) in 2023 propelled it into the spotlight, prompting increasing questions over how AI could be used to support the healthcare system. Deployed well and in the appropriate contexts, AI has the potential to help the healthcare system overcome a range of challenges.
With the field of AI evolving at pace, this primer has been developed to support healthcare organisations to navigate the noise. Intended for board members and wider teams, it demystifies the language of AI and showcases how organisations and systems are using it – and to what end. With more and more organisations exploring AI’s potential, this primer also suggests how you can use early conversations with suppliers to consider whether an AI solution is what you need.
Demystifying AI in healthcare: the jargon buster
Please note that not all definitions have an associated example as in many cases an example would be repeating the definition.
The basics
Interpreting models
Types of data
Types of machine learning
Types of model
Applications of AI
How the healthcare system is using AI
The potential uses of AI are widespread, from clinical uses to administrative support, in primary care settings and hospital settings. Below we have highlighted examples from within our membership that showcase the breadth of potential AI use within the healthcare system. These examples also put the AI jargon into a real-life context to help further understanding of key terminology.
Reducing DNAs and last-minute cancellations
Background
Mid and South Essex (MSE) NHS Foundation Trust supports a population of 1.2 million people. It had an average did-not-attend (DNA) and short-notice cancellation rate of 8 per cent (the average in England is approximately 7.6 per cent).
DNAs are more prominent in patients grappling with work and caring responsibilities, who find it difficult travelling to the hospital at a specific time. This disproportionately affects patients from minority and marginalised communities and can thereby lead to a widening of health inequalities. MSE found that its DNA rate for the most deprived was consistently higher than that for the least deprived across all age groups.
Previously
Many practices and hospitals send letters, emails, text and calls to remind patients of their appointments. These blanket approaches do not account for individual patient behaviours.
Healthcare staff may manually review appointment histories and contact patients who had missed appointments in the past, but this is time and resource intensive. Some organisations may overbook certain clinics to compensate for anticipated no shows.
Solution
MSE rolled out Deep Medical, which uses a model that integrates machine learning to predict patient no-shows and short-notice cancellations (<48 hours). Using AI, the system processes both structured data (like patient demographics and appointment history) and unstructured data (like electronic health record notes) to identify relevant patterns.
The predictive analytics model is developed through a deep learning model which is a subset of supervised machine learning. This model is trained using training data, fine-tuned with validation data and evaluated with the test data to ensure the model is accurate and precise and has high sensitivity and specificity. The neural network format of this model allows it to recognise complex patterns in real-world data.
It uses AI to understand patient engagement and develop personalised reminder schedules for patients. It then uses automation to send these reminders and manage patient appointments. It also identifies frail patients where low compliance could potentially be of clinical concern and highlights them to the relevant clinical teams.
Outcomes
Based on a six-month pilot scheme at MSE, the model:
- created an additional 1,910 patient visits into clinic
- prevented 377 DNAs
- filled 217 last-minute cancellation spots ️
- reduced DNA rate by up to 50 per cent when patients are proactively contacted two to three weeks ahead of their appointments
- when used at full scale, it is predicted it will allow an additional 80,000 patients to be seen each year at the trust, increasing productivity significantly
Top tips
Make sure you are clear on:
- what the specificity and sensitivity of the data is and what the impact of the mis-classified data is
- where the training data has come from and how valid it is in your local population or whether revalidation is required
- how to audit the machine learning to ensure it doesn’t drift from the primary use or introduce new biases.
Transforming wound care
Background
In North Cumbria approximately 50 per cent of the community nurses' workload involves managing both acute and chronic wounds, which amounts to the cost of around £41.7 million a year.
Previously
Prior to using the AI model, community nurses would assess wounds with a manual tape measure. Band 3 and 4 staff are sometimes unsure how to dress and treat more complex wounds, therefore, this would require taking a photo and sending it back to base where a band 6 or 7 would tell them how to treat the wound by looking at photos and advising how to change and apply the dressing remotely. In more severe cases the band 6/7 would need to go out to treat the wounds themselves, reducing time to care.
This was a very subjective way to treat and dress the wounds with a lot of room for interpretation and no consistency across community teams. There was no way to keep a record of the wounds and the changes.
Solution
North Cumbria Integrated Care (NCIC) Foundation Trust, as part of North East and North Cumbria (NENC) ICB, transformed its approach to wound care by introducing a digital tool, Minuteful for Wound by Healthy.io, that uses a model which uses an AI algorithm to support clinicians at various experience levels to assess wounds confidently, consistently and safely.
This model offers a higher degree of accuracy and precision than the current standard care. The digital tool enables consistent wound imagery, measurements and assessments to be captured easily at the point of care. The AI-powered colour recognition technology automatically detects wound area and tissue types within the wound bed from a three-second video scan of the wound by a smartphone. The AI also ensures image quality by recognising lighting conditions, distance and scan technique. Assessment prompts within the app guide allow clinicians of all experience levels to record assessments aligned to best practice guidance according to wound type.
The approach offers a live caseload review portal to provide central access to wound data. With this easily accessible data, clinical teams are able to optimise care plans.
The data gathered from AI measurements and assessments enables wounds that may be deteriorating or static over time to be identified and flagged for review that might otherwise not be identified. The new approach includes assigning clinicians to perform regular virtual caseload reviews within the tool's portal. This earlier detection and ability to review the caseload virtually, leads to earlier intervention and faster healing for patients.
Outcomes
Since using the model, NCIC has made the following positive impact for both staff and patients.
For the workforce, it has:
- opened access to data to help them understand wound care admission and case loads
- upskilled and retrained the workforce to dress and treat wounds with confidence, which has led to greater empowerment of junior clinical staff to undertake assessments
- provided assurance for staff as they are creating a log of the wound which provides detail on how the wound was treated if the patient is admitted into hospital
- released time to care for band 6/7 staff
- reduced admin burden for staff.
For patients, it has:
- reduced healing time and improved patient outcomes
- reduced hospital admissions
- supported self-care
- minimised the likelihood of future infections, admissions to hospital and amputations.
By working in partnership with the company, NENC ICB is hoping to:
- reduce incidents by early identification of deteriorating wounds and clinical risks
- standardise clinical data collection and reporting
- improve consistency of adherence to formulary and best-practice guidelines
- increase system-wide collaboration with ICS partners to expand digital wound management beyond the trust, to primary care, acute services and nursing/care home settings
- enable access to services, including emergency departments/vascular specialists both via app and the web page portal for greater information sharing.
Top tips
Make sure you are clear on:
- what IT infrastructure and resources are required for roll out and whether you have these already
- what training, standard operating procedures and ways of working are required for an effective roll out.
Impact on the workforce
To find out more about the benefits of this project for the workforce as well as patients, please read this case study.
Improving patient triage and optimising staff time
Background
Chapelford Medical Centre wanted to update its systems to triage patients in a smarter way, helping them get the care they need while also optimising use of staff time.
Previously
Chapelford Medical Centre had a triage tool and questionnaire to collate information that supported staff with triaging patients. This used automation and operated like a decision tree. The centre wanted to move to an approach that allowed evidenced-based and AI-supported decisions about the best course of action for a patient’s care. The ultimate goal was to make informed decisions and spot opportunities that would not be possible with a human-only approach, based on combining the information presented by the patient with information within patient records.
Solution
Anima, an integrated care platform, has both automation and AI modules. In the automation module, patients complete a questionnaire to support with triage and based on their answers, they will be directed to an appropriate care pathway.
In the AI module, Anima uses analysis of the structured and unstructured data input into the questionnaire by patients, and machine learning based on the outcomes of patients who go through the pathway to predict the best course of action for future patients. It uses a model and algorithm to follow the pathway of patients through triage and their outcomes, and refine the course of action for future patients with similar characteristics who fill in the questionnaire.
Outcomes
Anima went live within the GP practice in August 2023 and the ambition is to expand across the primary care network from August 2024.
The Chapelford Medical Centre team plans to be in contact with Anima regularly and provide mutual training support. The team will review and work out whether improvements can be made to the AI suggestion, and also improve their own experiential learning for next time by using a trusted source of information.
They are aiming to create an enhanced access system based on need, and not ‘first in the queue’. This includes better management of patients, ultimately less demand, an improved workload profile for clinicians, and a fully-functional multi-disciplinary team. AI will support the team at each stage, enabling staff to signpost effectively, and employ more junior team members to make effective and supported decisions. Access increases, patient need decreases, clinician workload levels out, and work-life balance improves.
Chapelford hopes to achieve a position where all patients get the care they need every time, first time. This is a challenge, but one that will significantly improve patient journey, and also maximise the potential of appointments by managing patients within one consultation. They aim to maximise individual clinician’s skills and achieve a 0 per cent need for referral between teams for the presenting condition.
Numbers of staff are unlikely to increase, so Chapelford hopes to increase effective capacity through more efficient use of the team and getting it right first time. By feeding back through an AI system, the system will continually improve and have a real-time feedback loop.
Top tips
Make sure you are clear on:
- which elements of the solution use AI and that it really is AI
- what the AI is improving or making better, and how it is measured
- what the clinical safety case is for the solution you are buying
- what your North Star is and whether the AI product feeds into a bigger and wider solution.
Transforming the cataract care pathway
Background
The cataract care pathway at Chelsea and Westminster Hospital was experiencing long waiting times and limited capacity,
Previously
The pathway required patients to attend a mandatory in-person pre-operative appointment before being placed on the surgery waiting list for their first eye. The did-not-attend (DNA) rate for these pre-operative clinics ranged from 30 per cent to 50 per cent, resulting in wasted clinical capacity.
Post surgery, although there are very low risks of complications and adverse outcomes from cataract surgery (less than 5 per cent), all post-operative patients were requested to attend an in-person appointment four weeks later. After consultation, patients were listed for their second eye surgery or discharged to the community.
Solution
Chelsea and Westminster Foundation Trust leveraged Ufonia’s Dora, an AI clinical assistant that can telephone patients and have a routine clinical conversation using AI-enabled automation, to transform their cataract care pathway.
Dora has a telephone-based voice conversation with patients at multiple timepoints in their cataract journey. This includes pre-operative assessment health screening, surgery and appointment reminders, post-operative checks and patient-reported outcome measures. The technology uses AI natural language processing to be able to interpret a patient's responses. It focuses on making AI accessible, meaning patients do not require any technology understanding, user accounts, hardware devices or training – they simply have a conversation.
Outcomes
Dora was initially deployed as a pilot, with pre-defined technical and operational success criteria. These were met during the initial phase meaning the trust now plans to adopt Dora to support their cataract patient pathways, advocate for regional implementation across North West London ICB, and is considering expanding the solution to other clinical pathways.
Successes include:
- 65 per cent call completion rate at pre-operative assessment stage, surpassing the original target of 60 per cent
- 91 per cent agreement rate between Dora and Chelsea and Westminster Hospital clinical staff, exceeding the goal of 90 per cent
- on-the-day cancellation rate has dropped significantly to just one patient in six months, compared to four patients per month before implementing Dora, surpassing the target of two patients per month.
- fewer than 2.5 per cent of patients at the post-operative check experienced an unexpected management change, well below our target of 10 per cent
- 63 per cent of completed calls passed the Dora assessment, exceeding the target of 50 per cent
- honoured as finalists in the HSJ Digital Awards 2024 for the Driving Change through AI and Automation Award.
Top tips
Make sure you are clear on:
- what implementation support exists from the tech side to ensure key milestones can be met
- what resources might be required and what lessons could be learned from previous implementations, to support internal resource allocation
- which metrics are being collected and when, to ensure robust evaluation following implementation.
To AI or not to AI? Key questions to consider
It is important to remember that AI may not be the appropriate solution to the problem your organisation is facing. Start with the challenge and ensure you select a solution that solves the issue. To support with innovation adoption, please refer to our Scaling Innovation guide.
To help determine whether AI is the right fit for solving the problem, and whether further exploration of the solution is required, we have prepared a set of key questions to ask suppliers of AI solutions in early discussions:
Questions to ask suppliers | What to look out for |
| Do the intended uses of the AI solve the issues you are trying to solve? What uses does the AI have regulatory approval and certifications for? Are there any exclusions or limitations you need to be aware of? |
2. What evidence is there supporting the effectiveness of the AI? Does evidence exist in real-world settings? | Has the solution been tested in a real-world clinical setting? How do the results compare to effectiveness without use of the AI component? Is the AI adding value? How similar is the training data cohort to your population? Are there potential biases that have been raised that you need to consider and explore in more depth? Does the supplier indicate if and how they mitigate against any biases? Has another organisation rolled out the product that you could get in contact with to gather their experience? |
3. What implementation support within your existing infrastructure is required? | What level of effort will be required to integrate the solution into existing digital pathways and for ongoing monitoring? Will any changes be required in IT and digital systems and data management? Has another organisation rolled out the product that you could get in contact with to gather their experience? |
These questions can be used to gather an understanding of the purpose of the proposed AI, the evidence supporting its use and the implementation support required.
If after hearing the responses you feel the product may be a good solution to the problem you are trying to solve, deeper exploration is required before a final procurement decision can be made.
Next steps if moving forward with an AI solution
Following the initial conversation, if you have decided the product could be a good solution, assemble a team of experts from within your organisation with expertise covering data and IT infrastructure, information governance, implementation and transformation, cyber security and clinical leadership (if the AI has a clinical component) to further explore the feasibility of adopting the AI into your organisation. There are several resources that can support AI adopters with navigating the complex environment of adopting AI within the NHS, including:
- A Buyer’s Guide to AI in Health and Care, developed by NHS Transformation Directorate in 2020 contains additional questions and detailed answers. The majority of the information is still relevant in the 2024 environment; however, elements of the regulatory environment section may not reflect the current situation.
- Developing Healthcare Workers’ Confidence in AI report developed by the NHS AI Lab and Health Education England in 2022 contains additional questions to consider when conversations with suppliers develop (pages 88-91).
- All Adopters' Guidance lists of all the guidance and regulations that apply to adopters of digital technologies in health and social care.
- Artificial Intelligence where the NHS Digital Academy has curated resources including a framework to support healthcare workers understand their level of understanding in AI.
- Adopt AI on the NHS England Transformation Directorate website curates a selection of resources.
If you are interested in sharing your experience of using AI within your organisation, or getting involved in future work the NHS Confederation is doing in this space, please contact: Rezina Hakim at rezina.hakim@nhsconfed.org